Unless you were living under a rock, you’d have heard of generative AI or Gen AI somewhere, sometime. Generative AI or Gen AI, a branch of artificial intelligence, has the remarkable ability to create new content that mimics human creativity. Imagine a digital artist, capable of composing music, generating artwork, or even crafting stories, all without human intervention.
This innovative technology operates on complex algorithms that enable machines to learn patterns from vast amounts of data and produce original outputs. Just like a skilled storyteller creating stories from imagination, generative AI crafts narratives, designs, and music in an impressive time frame.
Key Takeaways
- Generative AI (Gen AI) uses advanced algorithms like GANs to create diverse, human-like, and original content.
- Unlike traditional AI, it learns from vast datasets to produce lifelike images, text, and music.
- It also raises challenges such as ensuring content quality and addressing ethical concerns.
- Raises concerns of job displacement and the devaluation of human-created content.
What is Generative AI?
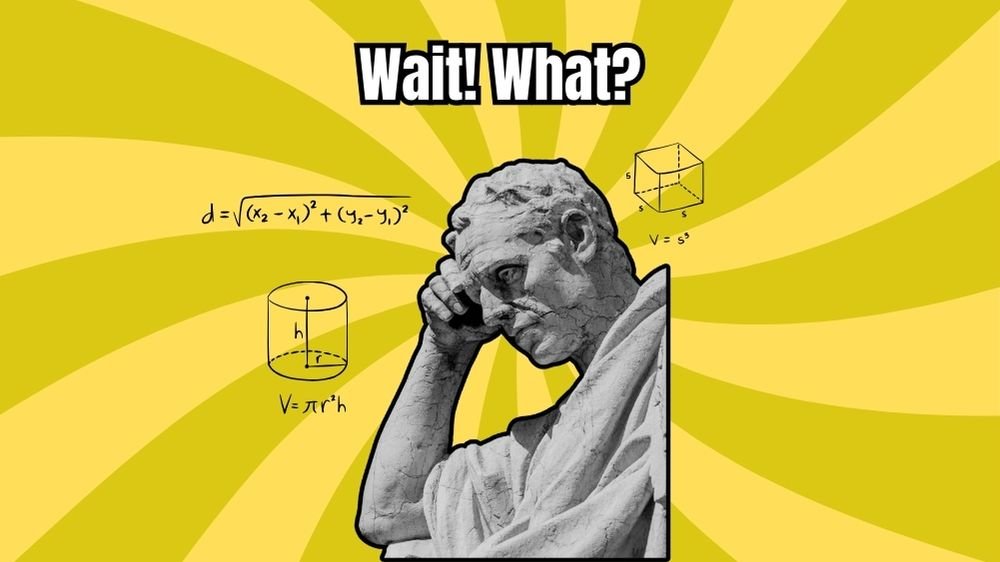
Gen AI is a special kind of AI that’s all about being creative. While regular AI tackles problems in a specific way, Gen AI goes beyond that and gets into making new stuff up. It uses something called Generative Adversarial Networks (GANs) to come up with things like pictures, writing, or music that looks like it could’ve been made by a person.
All this happens through a process of learning from vast datasets. Gen AI models develop the ability to synthesise original content. From generating artwork and music compositions to aiding in content creation and data augmentation, Gen AI holds immense potential in revolutionising how we interact with technology.
You know how regular AI is like a really smart calculator, right? It’s great at solving math problems and puzzles, but that’s pretty much all it does. Now, imagine Gen AI as a whole new kind of smart robot. Instead of just doing math, it’s like an artist! It can make up its own pictures, stories, and music. While regular AI follows strict rules, Gen AI gets to be more like a creative thinker, coming up with its own ideas.
Gen AI vs Traditional AI
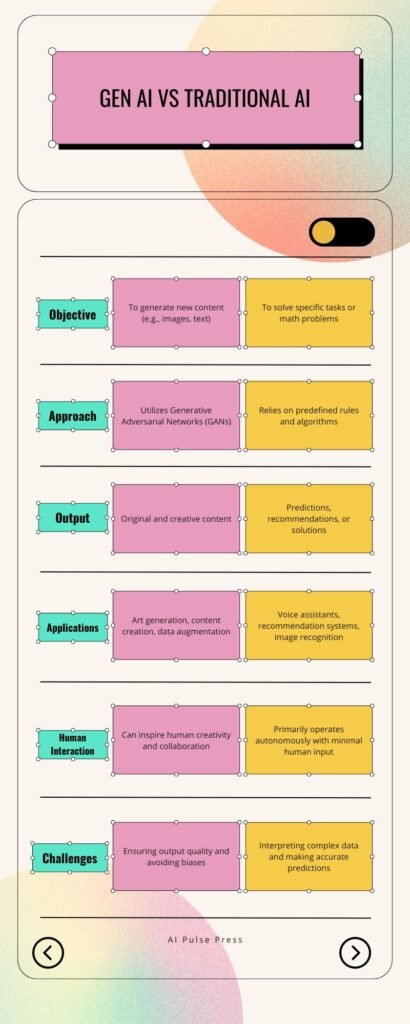
How Does Generative AI Work?
Generative AI uses smart algorithms to make new stuff that looks like humans made it. It works by using a technique called Generative Adversarial Networks (GANs), where two computer networks, the Generator and the Discriminator, kind of compete.
The Generator makes things like pictures or text by learning from big sets of data. Then, the Discriminator checks if what the Generator made looks real or fake. They keep going back and forth like this, getting better each time.
For example, if they’re trained on human faces, at first, the Generator might make blurry or weird faces. But with feedback from the Discriminator, it learns to make better, more realistic faces. This process helps Generative AI create more and more lifelike stuff as it goes along.
Generative AI finds applications in various domains, from art generation to content creation and even data augmentation. For example, in the field of art, Generative AI can create original paintings or sculptures, inspiring new forms of artistic expression. In content creation, it can generate realistic text, music compositions, or even entire stories. Moreover, in data augmentation, Generative AI can generate synthetic data to supplement existing datasets, enhancing the performance of machine learning models.
Despite its capabilities, Generative AI faces challenges such as ensuring the quality and authenticity of generated content and avoiding biases present in the training data. Additionally, as Generative AI becomes more sophisticated, there are ethical considerations regarding the potential misuse of generated content, such as deepfakes or misinformation.
Looking ahead, Generative AI holds immense potential to revolutionize various industries and bring innovation. By enabling machines to generate creative and original content, Generative AI opens up new possibilities for human-machine collaboration and creative exploration.
Will Generative AI Replace Humans in the Workplace?
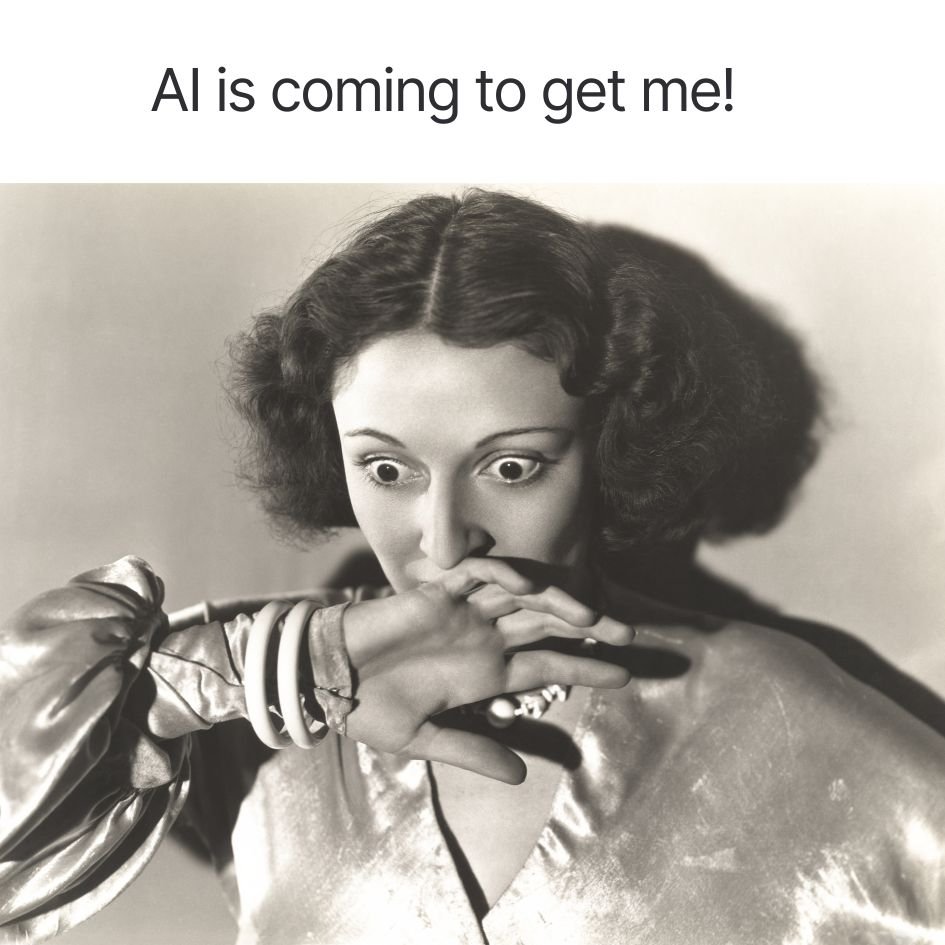
Generative AI presents a paradigm shift in the workplace, sparking debates on its potential impact. While advocates argue that it will create new job opportunities alongside automation, skeptics raise concerns about its implications for human-created content.
AI advocates stress that Generative AI complements human efforts, requiring human intervention in data selection and model evaluation. However, critics fear a devaluation of human-generated content as Generative AI becomes proficient in imitating various styles.
Notably, the recent writer’s strike in Hollywood raised these concerns. As studios turned to AI-powered writing tools for content generation and editing, writers voiced apprehensions about job displacement and content quality erosion.
The rise of Generative AI heralds both opportunities and challenges in the workplace. While it promises innovation and efficiency, concerns about job displacement and content devaluation linger. By fostering dialogue, establishing guidelines, and upholding human creativity’s intrinsic value, we can harness the potential of Generative AI while safeguarding the integrity of human ingenuity.